Performance: Each 0.1 Second Is Worth $18M For Bing
Every millisecond decides whether a user will stay or leave Bing. In this session, hear from Ronny himself how Bing ensures a great experience to its users.
Transcription
0:06
Ashwin: Hi! Welcome to ConvEx where we are celebrating experiment driven marketing. I’m Ashwin and I handle growth at VWO. Want to fix leaks in your conversion funnel? Try VWO. I’m excited to have Ronny Kohavi with us who is Corporate Vice President Analysis and Experimentation at Microsoft. Glad to host you Ronny!
0:27
Ronny: Hello Hello. Glad to be here.
Ashwin: So before I pass the mic to Ronny, I would want to inform you that you can join ConvEx’s official networking group on LinkedIn and ask your questions from this presentation. With that Ronny, the stage is all yours.
0:44
Ronny: Thank you very much. I’m going to talk about performance, and the key result that I’m going to share is one of those quantifiable things that’s easy to understand – ‘Each 0.1 seconds for Bing is worth 18 million dollars.’
1:04
This of course is a clear quantifiable result. We’re going to talk about some other things that improved as we ran the experiment to assess the value of performance. So let me start with some motivation – Steve Souders said it really well in his book, “The dangers of a slow website: frustrated users, negative brand perception, increased operating expenses, and loss of revenue.”
We are going to show you through controlled experiments how we were able to quantify these areas and more important we came up with the return on investment equation, which is: how important is performance for us at Bing and on other properties.
1:49
Should we put a full-time person on performance, maybe 5 people maybe 20 people. That equation that you’re able to get from an A/B test or controlled experiments on performance is critical for deciding how to allocate resources. So the key is you want to inform the HiPPO (i.e Highest Paid Person’s Opinion) with data about the importance of performance. Key questions are – what metrics are being impacted? So I shared with you one of them which is revenue – an easy one to quantify. But there’s many other things that improved with performance and we’ll go through those. And another key benefit of this experiment is that if you’re implementing a new idea and let’s say the first implementation is inefficient and happens to degrade performance by, let’s say, 50 milliseconds. What can you expect [from] metrics if you were to re-implement this idea more efficiently and save back those 50 or maybe 45 out of those. The ability to run an experiment and quantify the relationship between performance and key metrics is fundamental for many many things. So, let me start with a punch-line.
3:03
As I mentioned in the title of my talk, we’re quantifying a hundred milliseconds degradation or improvement for key metrics. In addition to revenue, success rate improved a lot. And how do we define success? Success is defined as a click from the Bing search engine results page to some destination – so you see the result the user clicks through. If they don’t come back quickly within 20 seconds, we consider it to be a successful click, i.e., we found some destination that they’re interested enough to stay longer than the 20 seconds. If they come back quickly, then it’s considered to be sort of a click bait. It’s probably a website that isn’t good or has a problem so the user comes back from it quickly. So in this experiment that we ran, we were able to see that one of our key metrics, success rate, improved.
3:59
How long it takes to reach that successful click that also improved. Meaning it was lower, which is a good thing to do. And then finally the revenue impact improved by 0.6% for that hundred millisecond. At the time Bing was 3 billion dollar business now it’s worth a lot more. So if you just think about it, 3 billion dollar business 0.6% that was 18 million dollars a year. That’s a lot of money for a hundred milliseconds. For many ideas that we have, there’s a clear trade-off between the revenue and user metrics like the ones I’ve shown above. The nice thing about the performance improvement is that effectively everything improves, which is something very rare.
4:47
So I want to start off by saying how we got in here. How do we get to even look at performance? And there’s a kind of simple nice story that happened. Somebody ran an experiment where they were able to shrink the size of the page or the HTML (it’s called page weight) and performance improved and then we saw a lot of other metrics including revenue, improved. And it improved by more than [what] we expected. So we were wondering if there’s something unique to that and that’s what led for us to run this experiment that isolates just the performance. So very important to do that. The results are not unique to Bing. Amazon ran an experiment where they slowed down the website by a hundred millisecond and were able to measure the speed up if it were a hundred [millisecond] (we’ll talk about that later) and they concluded that there’s an impact to one percent of gross merchandising sales. Google also ran experiments on performance and you can see here a talk by two people one from Bing and one from Google sharing some of the key metrics that were impacted at search engines.
5:57
Okay, so I’ve shared with you some cool results. How do we arrive at these? So I just want to say one of the key things is life is messy and arriving at these conclusions is not easy. This requires some assumptions. We’re going to walk you through them to see that what we had to arrive in order to design the experiment, and what we had to assume for that to happen.
6:24
So what I’ll describe is the end-to-end controlled experiment or an A/B test, its design, execution, and I’ll explain why the assumptions that we made are reasonable, hopefully convincing you that this result holds. With that I want to pause for maybe a minute, take you through the description of a controlled experiment. I think this audience is likely to know most of this but let’s just make sure everything is set. So you’ve got some users, you’re going to split them into two groups – some go to the control which is the existing experience, some go to the treatment which has some change. And our experiment will show you what we did in order to do that.
7:04
The user’s interactions are instrumented – meaning we record the user’s click, how long they stay on the page, hovers, things like that all are reported to the server. And then at the end we have to analyze the experiment to see if the differences are material. So a few things to note: one is we’re running these experiments on real users; these are not beta testers, these are not insiders. These are the actual users that use the product and that’s pretty important because what you want to do is generalize and say ‘after this experiment when I ship to a hundred percent this is what’s going to happen.’ The second thing to realize is that you must conduct statistical tests. Here at the bottom when you analyze the experiment, because you are splitting the population and due to random fluctuations, due to chance it is possible that there are some users that are different on the left-hand side and the right-hand side. So by running statistical tests, you can see if the differences are unlikely to be due to chance.
8:11
Controlled experiments – they’re considered to be the gold standard the best scientific way to prove causality. And this is the key word here. The changes that we see at the bottom are caused by this change that we introduced in the treatment with high probability. And that’s the key reason why control experiments are for example, the only way to approve drugs in the US – the FDA requires you to run a randomized clinical trial. In the software world, it’s much easier, we can run hundreds of these, and this is the way for us to assess what happened with the treatment and what metrics it caused to be different. So let’s look at the crux of the experimental design. Most ideas that we have to speed up to impact the performance, impact some other factor, i.e, we can reduce relevance and do it faster, we can remove some page components and serve a lighter page. But this conflates multiple factors. What we would like to do is isolate just the performance.
9:24
But if we knew how to speed up the performance without hurting other factors, we would have done that yesterday, right? So this is something hard to do. The solution, therefore that we use is to slow down the website or product instead of speeding it up. It’s easy to slow down but it requires an assumption. So let’s look at how strong or weak this assumption is.
9:48
So the key assumption that we make is that near the measurement point, the degradation impact is about the same as the improvement impact. So let’s look at the graph below and see what this assumption means.
We’re looking at a graph of page load time on the x-axis, how long it takes to load the page, relative to some metric, say revenue. And the graph looks something like this:
The faster you go, the more you move on the x axis to the left, the likely it is that some metric improve whether it is success rates, time to success is going to be lower so the graph will look this way or revenue. We are here at a certain point on the x-axis and instead of moving left, which we find hard to do, we’re going to move to the right and the metric will therefore change by this amount. The assumption that we make is that had we moved left the difference on the left would be similar to what we’re observing on the right.
10:58
Okay, so this is typically called the first order Taylor series approximation or linear approximation effectively saying that near this point if we had a straight line,then this delta would be similar to this delta. Seems like a reasonable approximation. But in order to test it, we actually slowed down the website at 2 points here and then here. We slowed it down by a 100 millisecond and by 250 milliseconds and we measured that those deltas that we observe are pretty much close to a straight line and that allows us to validate the assumption.
11:49
How much should we slow down? So there’s a trade-off here as in many things and experimentation if you slow down a lot, it hurts the user experience. For the duration of the experiment if we slow the users too much, we are giving them a poor experience. However, if we slow down too little it is hard for us to estimate the relative impact because of the confidence interval. So let’s look at the graph below: we could slow down by a hundred millisecond and we would have this wide confidence interval that would be between this high point and the slope point. In fact, in this example, (which is not the real example) it even crosses the zero line, which means that this result is not statistically significant. But if we slow down more and we’re able to move here, then this confidence interval is higher and allows us to better assess the slope or the slowdown of what happened to the metric. Therefore, what we decided to do after a few trials was to hone in and run the experiment at a hundred and two hundred fifty milliseconds or quarter of a second.
12:56
Okay, I want to do a small leg rush into a technical point because I think it’s interesting to see how complex things get in some of these experiments. We ran this experiment which was multiple years ago, we had to estimate the page load time on the clients browser, with a pretty fancy technique. The purpose of the next slide is just to show you this nice trick that we use in practice. It is useful in some of the older browsers. It is also very detailed so if you don’t get it, don’t worry about it. It’s not germane to the final crux. It is important for some people to know how to measure page load time when you can’t do it on some of the newer browsers and the reason it’s less important today because the newer W3C standards support what are called navigation calls, and they allow you to call and ask what the load time was. But let’s look at what happens when this is not available.
13:52
So let’s start explaining this graph: you have here the client time (and I’m pointing to this line at the bottom), and the server time at the top. The user starts at T0 – they initiate a request, they type a query, they hit magnifying glass, or hit return and some time elapses until the server gets this query.
Now, we’re at T1: the server receives the query. The first thing that the server tries to do is to start sending some HTML back. And this is something important, we’ll talk about it later. But this – by sending some results, the page erases on the client the frame, or the Chrome of the search results starts to paint, and that gives the user experience that something is happening. Sending that HTML take some time.
14:46
So now the client is at T3, but the server side continues to run, moves along, figures out what the page should look like, what are those 10 blue links or other information, adds everything else, sends that to the client again. The client may do a bunch of requests to receive images or other things and at some point it fires what is called the onload event. The onload event says that the browser is ready, the page is now displayed. We send at this point a beacon to the server saying the onload event fires.
So all this complexity and what are we really looking at? We are trying to measure the time T0 to T6 (this is the page load time.) But we can’t measure it directly. So what we’re going to do is we’re going to use T7 on the server minus T1 on the server as an approximation for the page load time. So we’re now approximating the page load time and we’re doing it on the server because clients clock or less reliable, and because this time of T0 is not available on the earlier browsers. Now is this assumption reasonable? We think it is and the reason is that this time that it takes between T0 and T1 is very similar to the time between T6 and T7. In the first one the request with the query goes to the server, in the second one the web beacon or small one by one pixel is being sent to the server. Both of these are small request, the time that it takes for the network for the request to reach on the network from the client to the server is likely to be the same. And with the newer standards we’ve been able to validate that this technique to approximate page load time is very reliable and it works on all the browsers that are reliable.
16:49
Okay, so everything is ready.
16:54
We started running the experiment and what we did is we delayed the server responds by a hundred millisecond in one treatment and 250 milliseconds in another treatment.
17:05
But the impact was great. It was so great that we invoked what we commonly call Twyman’s law, ‘any figure that looks interesting or different is usually wrong.’ You know, I love to think of data scientists as skeptics; when you get a result that is extreme, double and triple check things. And indeed we decided at this point is that we ran the experiment the wrong way.
17:34
Let me leave it for a few seconds for you guys to think. What was wrong with our initial implementation of slowing the server response by a hundred milliseconds or 250 milliseconds and the two treatments?
18:03
So what we decided is that we slow down chunk one, we slow down the whole server. Remember chunk one is this first server response. It is query independent. It doesn’t matter what you typed in the query, we send a bunch of HTML to start painting the page to put the newer search box, to put the frame, to put everything around it. It’s very important that this chunk one gets to the client extremely quickly. And indeed we put a lot of effort to send it really quickly from the server – nothing needs to be computed. Any query that you do we’re just going to send that response that we know what it looks like, i.e., the initial HTML and additional JavaScript, because this is dead time effectively – the time server is using to compute the rest of the page, those 10 blue links. That chunk 1 gives the user a signal that the query was received and that the rest of the page is coming soon.
19:01
It’s also very hard to improve the time to chunk 1. Remember the server responds really quickly when it receives this request. So it’s unlikely that anything we do is going to allow us to improve the time it takes the chunk one. It’s therefore we decided that this initial treatment was not going to represent what we really wanted to test. What we really want to do is inject delay after chunk 1, but before the next chunk that actually haults the results is being sent. Okay, so we made this change. Now, it’s always good to validate your assumptions.
19:38
We inserted a delay, let’s say 250 milliseconds. Did we see that delay materialize? Well, not exactly. And again, this is real life, it’s messy. For example on the 250 milliseconds treatment delay, we saw the page load time delay by only 236 millisecond – close, not exact. Why is it not exact? We have millions of users in the experiment things should be exact. Well, they’re not. More interestingly that delay if you break down by browser was actually different.
20:15
And so we really have to work on this and figure out the reason. One reason was that sometimes the server is not ready to send the next chunks. Those contain images or other things and it takes time to compute them. The delay effectively makes the rest of the chunk be ready. So there’s a little bit of extra time that we saved by waiting and so it was little under 250. The other interesting thing is that all their browsers were so slow to parse chunk one which contains a bunch of JavaScript that in some cases they weren’t even ready to receive chunk too. Adding that delay gave them that extra time but we really didn’t save all the 250. We didn’t really add the 250 milliseconds. So is this reasonable? Yeah, the world is messy, we decided this was close enough. And if anything, our impact assessment is a little bit conservative because we assumed it was 250, when in reality, it was less by a little bit, a little bit more for the older browsers.
21:14
A few things to end here: one is the perceived performance is really what matters. Our experiment slow down the server’s response for the search results page key elements that is the algorithmic results in the main line adds. Other page elements are much less important. So we ran another experiment where we slow down that right pane. We slowed it by the same 250 milliseconds and we could not observe any significant impact. So another example if you slow and delay elements below the fold that is (where the user is visible) what the user sees in their current window, users are unlikely to notice it unless they scroll fast.
21:57
So this is another trick if you can compute the stuff above the fold really quickly and delay stuff below the fold, you are likely to gain a lot of value.
22:10
And then the final key point is that it’s very important to realize that the metrics that we show, those punchline metrics, are relative to today’s perf. So that 0.6 percent that we showed revenue improve, that’s (relevant or) relative to where we are today. It quantifies what the impact is in the control experiment relative to where we are today in performance. If we improve the performance over time that impact may change. In fact, it was interesting our initial run was done in 2012 when Bing’s performance was about one and a half seconds at the 90th percentile. Several years later when we re-run the experiment the performance are already sub-second.
22:53
Is it still important to improve performance or have we reached a point where it doesn’t matter much for the users? Interestingly, (we found) we re-ran the experiment and found that indeed the performance impact was not as severe. It was about 20% less relative improvement for many metrics including revenue. However for metric like revenue, oh an important point, reducing by a hundred millisecond becomes a lot harder when you’re below 1 second than where we were second and a half. What we found is revenue at that time was three times higher than it was in 2012. So the monetization impact of a hundred millisecond was only 80 percent, but it was on a base three times higher. So the improvement factor was actually 2.4 times bigger.
23:46
So to summarize one of the things that I like is to be able to share with users something that’s memorable. And this is something that we used after our first experiments in 2012. We told people if you’re an engineer and you’re able to improve the server performance by 10 milliseconds, which is much faster, 1/30th of the speed that your eyes blink. You just paid for your fully loaded annual costs at Microsoft and that includes everything fully loaded and that includes the fact that revenue has to be translated into profit by multiplying by the margin. Every millisecond counts. What happens if few years later, I told you that we re-ran the experiment?
24:31
Well, the 10 millisecond was updated to 4. If you were able to improve the performance by just 4 milliseconds, you now paid for your fully loaded annual costs.
24:45
Thank you.
Ashwin Gupta: Awesome. I had one question I was really interested in asking you because you said over a period of time, you know, you’re reducing the performance impact. As you reduce it, it requires more effort to actually get that going. So at what point do you kind of figure out the cost versus output debate, that you should probably let go of performance and focus on something else, right?
Ronny: It’s a really great question. So what I said in one of the earlier slides is there’s this return on investment equation, which is what does it take for you to reduce by a certain number of milliseconds? And what is the value that you get? And we’ve made this equation very explicit. Now, what we do today is two things, one, you’re absolutely right, it’s much harder to improve the performance that we are at, but because we keep introducing features those features need more time. And so we have this sort of an accounting mechanism where there’s a group that works on improving the performance and they put the money or the savings in the banks, and the other groups are given some of that performance improvement cash to be able to use to improve relevance or other algorithms. So yeah, it’s a really good question and there’s a really good answer to it which is yes, we’re very careful about the ROI. We want to make sure that we don’t slow things down when somebody comes up with a new feature they say – look at my go feature – and it slows down the side by 5 milliseconds.
26:12
We’re like, hey, are you willing to give up a person on your team? Because that’s what it’s going to cost us. So there’s this trade-off that we always have to make sure that we are improving the features. And indeed may be the initial implementation is slow just to look at the idea and we are able to adjust to say, we were able to make it faster, this is how much we would gain back, and then the future implementation, the later implementations have to be much more efficient.
Ashwin Gupta: Right and you also talked about like there’s a delta across browsers that you saw and what you were expecting to reduce the page load time by was not the case because people are on different browsers, different performance? So do you also look at like different devices and browsers and different internet speeds and focus on that aspect? So maybe not for the entire thing but optimizing for these chunks.
Ronny: Absolutely. So whenever somebody runs an experiment we produce them what is called the scorecard with lots of metrics. But the other thing that we do is we’re able to allow them to segment by different attributes of our user base – by browsers, by data centers that we sometimes need to look at, by tenure of the user population – some newer users may be impacted more than older users, by the type of queries that they do. So there’s a lot of segments that you can look at. One of the cool features that we implemented is that we automatically have implemented some machine learning algorithms. They’re able to point out that ‘hey here in this specific segment there is a significant change to some metric go look at that (called segments of interest). ‘
Ashwin Gupta: That’s great. So you’ve been running controlled experiments of Microsoft for almost 14 years. So what has been a key lesson from an experimentation standpoint from Microsoft? I would love to hear it from you.
28:05
Ronny: Yeah, so I think one of the things that is really important is to build a system that encourages running experiments correctly – meaning trustworthy experiments and we put that in our early mission statement that we want to accelerate innovation using trustworthy experimentation. And so for example, when you run a test, we do a set of checks. One of them is called the Sample Ratio Mismatch. If you were going to run an experiment at an equal percentage from control and treatment did you actually get approximately the same number of users in control and treatment? So there’s a few papers out there that describe why these SRMs happen, what are the reasons and when they fail, when that SRM check fails, what you normally see is very extreme result either very good or very bad. So what you learn is really make sure to run those tests, and two when you do get an extreme result invoke Twyman’s law – it’s extreme, there’s probably a bug in there someplace.
29:07
Ashwin Gupta: Make sense. And Microsoft has been running experiments for a long time. So I would love to know what is the experimentation culture at Microsoft, how do you approach experimentation, how it kind of plays a role in how you shape up your products because Microsoft is almost everywhere. So would love to understand the role experimentation plays in your product.
Ronny: Yeah, great question! So the experiment took off at Bing where we started to look at the data seriously and make sure that every feature that is written is being evaluated with controlled experiments. Given the success of Bing, it was clear that we should take this to other parts of the company and I’m proud to say that over the last few years every major product that Microsoft is now using experimentation. Whether it’s MSN or office or Xbox or Windows, the browser all these groups are now using experimentation and learning more and more that we have to be agile, ship often, listen to the users, get the metrics, understand the impact. Because normally we’re too optimistic about the value that we think our new ideas have, every new feature, and the new shop and shiny object that we’re sure is going to be the greatest thing since sliced bread, but it turns out one of the roles, and we always laugh about this, one of the roles of my team is to actually give you the data and sometimes tells you that your new baby is ugly.
Ashwin Gupta: Well, I think you enjoy that. All right. Yeah.
Ronny: I’ll just share one more interesting insight which is ‘most ideas fail.’ And this is a very humbling thing. One of the things that we found is that it’s about ⅓, ⅓, ⅓, : about a third of our ideas are useful and statistically significantly better, about a third do nothing – you thought it would be good, it does really nothing – and the surprising part is that a third (⅓) are actually negative – it may be bugs in the implementation, it may be that the idea is bad. but it’s a very humbling number. And in fact in groups, like Bing that optimized for a long time the failure rate is actually higher. About 80% of our ideas are flat or negative. Very hard to come by new ideas that are breakthroughs.
Ashwin Gupta: Oh wow! Very interesting insight. So alright so I think I’ve asked the questions, but I would kind of offset the conversation I would love to understand or know about a book that you’re reading right now. And yeah some interesting nuggets from it.
Ronny: Yeah. Let me answer this in two ways: one is I’m writing a book. I’m finishing a book on experimentation. We just handed it a couple of weeks ago to the publisher. So it should be out in about three months. If you’re interested, you can go to exp-platform.com and there’s a section there called Advanced Topics and experimentation with the draft book. I love reading about areas that are sort of data enforced and one of the books that I’m reading now is called Dying For A Paycheck by Jeffrey Pfeffer. I found these at Stanford, I found that the guy is able to write books that not only are written well, but he also brings in data from a lot of scientific research. So it’s not a technical book as much as a cultural book about how some of the things that we do on organization, some of the stress that we put is actually hurting us in the long run.
Ashwin Gupta: Awesome. I would love to know a bit more about the book that you are writing. And when is it coming out? Would love to know about that.
Ronny: As I said, we just handed it to a publisher and hopefully in about three months. So somewhere around October/November, you’ll be able to see it on Amazon and other places.
Ashwin Gupta:Awesome. That’s exciting. We’ll leave a link in the talk for that. So yes, I think that’s it from my side. It was a great conversation, and I would love to get my audience to connect with you.
Ronny: Yeah. So the best way is to look at the site exp-platform.com. It has contact information there, it has papers that we wrote. You can follow me on Twitter, its @RonnyK, and connect with me on LinkedIn – all these are fine methods.
Ashwin Gupta: Awesome. All right, Ronny. Thank you so much for this presentation.
Ronny: Thanks for inviting me. It was my pleasure.
Other Suggested Sessions
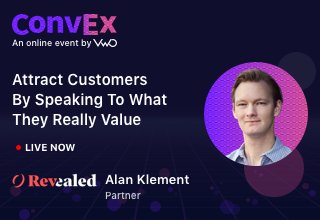
Attract Customers By Speaking To What They Really Value

This presentation will walk you through a case study of how a company applied JTBD theory to understand what consumers really wanted.